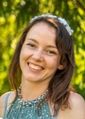
Christina Bornberg
University of Girona, Spain
Title: A mean-shift approach for diverse example understanding in semi-supervised drusen segmentation
Biography
Biography: Christina Bornberg
Abstract
Replacing routine diagnosis tasks such as drusen detection in the medical field with deep learning approaches such as convolutional neural networks (CNNs) should lower the workload of medical doctors. To train such a CNN, labels are required that need to be obtained by a medical doctor. This task is time con- suming and therefore research in fields such as semi-supervised, self-supervised and unsupervised learning has been made, where little to no labels are needed. Semi-supervised learning generally uses a dataset where only a small number of images are labelled. In this ongoing work, we want to understand what similar- ity in an image means according to convolutional neural networks to determine which images should be annotated in a dataset and reduce the time needed by a medical expert to label images. The approach that is currently under develop- ment is a combined clustering and knowledge distillation approach to determine memorable examples. First, a feature vector is obtained from a pre-trained classification network for each image. Principal component analysis (PCA) is applied for acquiring more meaningful and reduced features.These features are fed into a mean shift clustering implementation, which produces clusters based on density. Furthermore, we want to perform classification based on the clusters to figure out the memorability of images. To evaluate the approach, we want to perform segmentation with a semi-supervised approach where labels are only used for memorable examples while the remaining images are used without labels. This will be compared to a fully supervised approach.